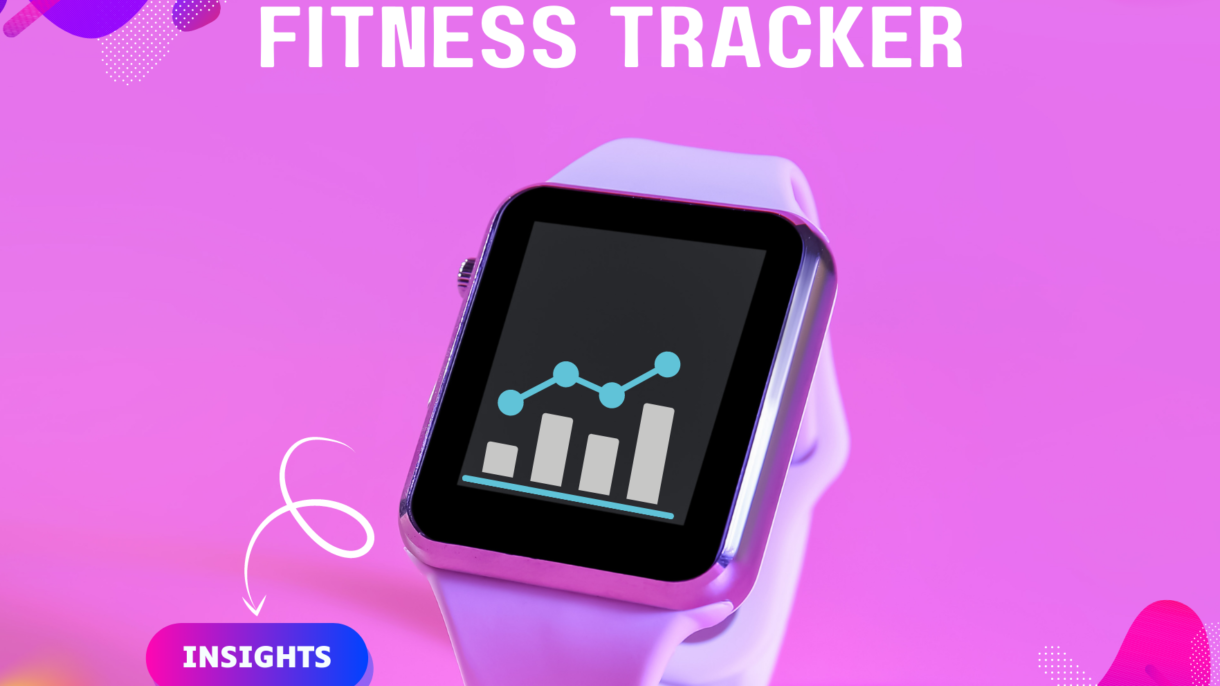
Fitness Tracker Market Analysis
Get in shape for success with Fitness Tracker Market Analysis – your guide to staying ahead of the competition!
– Understanding Buying Patterns and Price Drivers in India
As a fitness enthusiast, I embarked on a project to analyze the buying pattern for fitness trackers in the Indian market and determine what factors drive their prices. My aim was to gain insight into the Indian fitness band buying population and help companies understand the variables that affect the overall sales of Fitness bands.
To achieve my goal, I gathered my dataset from Kaggle.com, which I had originally collected from an e-commerce website Flipkart. I was confident that my sample accurately represents the Indian fitness band buying population as it was randomly selected.
After analyzing the dataset, I found that the selling price of Fitness watches is based on various variables like Original Price, Rating, Average Battery life, and FitnessBand. I observed that the average cost for Apple products was the highest, followed by Garmin and Samsung. However, Apple had the least battery life, while Garmin had the most battery life.
In the second part of my project, I aimed to determine how various factors like the Original Price, Display, Average battery life, Rating, Color, and Device type affect the selling price (dependent variable) for a Fitness watch. To achieve this, I developed a regression model with maximum predictive power to evaluate the relationship between the dependent and explanatory variables.
I used scatter plots to determine the association between the X and Y variables. My dependent variable was the Selling Price, and the explanatory variables were the Original Price, Rating, Average battery life, Display, Color, and Device type. I assumed the Original price to have a linear relationship with Selling Price because Selling Price is obtained from Original price. I calculated dummy variables for Color, Device type, and Display to convert categorical variables into numerical variables.
I selected Average Battery Life and Rating to predict their effect on Selling Price. Based on the regression analysis, I concluded that using the equation for Predicted Selling price, I could accurately predict the selling price of a Fitness Band with 96.7% accuracy.
My project’s findings will help companies that produce fitness products effectively predict the selling prices of their products and understand how variables like average battery life, original price, ratings, Display, Color, and Device type affect the overall sales of Fitness bands.